Image classification: Difference between revisions
(+ A SMAP Supervised Classification of Landsat Images for Urban Sprawl Evaluation, 2016) |
mNo edit summary |
||
Line 284: | Line 284: | ||
== Further reading classification with GRASS == | == Further reading classification with GRASS == | ||
* Micha Silver: [ | * Micha Silver: [https://www.surfaces.co.il/analyzing-acacia-tree-health-in-the-arava-with-grass-gis/ Analyzing acacia tree health in the Arava with GRASS GIS] | ||
* Perrygeo: [http://www.perrygeo.net/wordpress/?p=104 Impervious surface deliniation with GRASS] | * Perrygeo: [http://www.perrygeo.net/wordpress/?p=104 Impervious surface deliniation with GRASS] | ||
* Dylan Beaudette: [http://casoilresource.lawr.ucdavis.edu/drupal/node/159 Working with Landsat Data] | * Dylan Beaudette: [http://casoilresource.lawr.ucdavis.edu/drupal/node/159 Working with Landsat Data] |
Revision as of 13:46, 5 August 2016
Image classification
Classification methods in GRASS
radiometric unsupervised |
radiometric supervised 1 |
radiometric supervised 2 |
radiometric & geometric supervised | |
Image Preprocessing | r.smooth.seg (optional, in case of noisy original data) | |||
Segmentation | i.segment | |||
Preprocessing | i.cluster | i.class (monitor digitizing), g.gui.iclass (in GRASS 70) | i.gensig (using training maps) | i.gensigset (using training maps) |
Classification | i.maxlik | i.maxlik | i.maxlik | i.smap |
Remarks | automated run based | requires digitalization | requires digitalization | requires digitalization |
on image statistics | of training areas | of training areas | of training areas |
You can digitize training areas with either r.digit (not recommended) or v.digit GRASS Digitizing tool + v.to.rast (recommended)
Image Preprocessing (optional)
- r.smooth.seg - produces a smooth approximation of the data and performs discontinuity detection. The module is based on the Mumford-Shah variational model for image segmentation. Used as pre-analysis for a subsequent classification.
- For details, see the r.smooth.seg manual (formerly called: r.seg)
- i.segment - identifies segments (objects) from imagery data based (currently) on a region growing and merging algorithm. The image segmentation results can be useful (on their own or) as a preprocessing step for image classification, i.e. reduce noise and speed up the classification.
- Classification of these segments: see below in "Unsupervised classification".
Interactive setup
- i.class - Generates spectral signatures for an image by allowing the user to outline regions of interest.
- The resulting signature file can be used as input for i.maxlik or as a seed signature file for i.cluster.
- g.gui.iclass - Tool for supervised classification of imagery data.
Preprocessing
- i.cluster - Generates spectral signatures for land cover types in an image using a clustering algorithm.
- The resulting signature file is used as input for i.maxlik, to generate an unsupervised image classification.
- i.gensig - Generates statistics for i.maxlik from raster map layer.
- i.gensigset - Generate statistics for i.smap from raster map layer.
Background information concerning i.cluster:
Unsupervised classification
For an introduction, see for example Cluster_analysis.
- i.maxlik - Classifies the cell spectral reflectances in imagery data.
- Classification is based on the spectral signature information generated by i.cluster (see above)
- GRASS GIS 7: i.segment
- Classification of these segments could be achieved with these addons: v.class.mlR, v.class.mlpy, v.class.ml.
See example below.
Supervised classification
- i.maxlik - Classifies the cell spectral reflectances in imagery data.
- Classification is based on the spectral signature information generated by either i.class, or i.gensig.
- g.gui.iclass - Tool for supervised classification of imagery data.
- i.smap - Performs contextual (image segmentation) image classification using sequential maximum a posteriori (SMAP) estimation.
- In case of panchromatic maps or limited amount of channels, it is often recommended to generate synthetic channels through texture analysis (r.texture)
See example below.
Small classification tutorial
Required: GRASS >= 6.4
We are using Landsat satellite data prepared for the North Carolina sampling site. It is a multispectral dataset where each channel is stored in a separate map.
We are using the North Carolina location here.
Warming up with Landsat TM
# look what maps are there g.list rast # set to one of the color channels and print current region settings g.region rast=lsat7_2002_10 -p
# look at some Landsat map (1: blue, 2: green, 3: red, 4: near infrared etc.) d.mon x0 d.rast lsat7_2002_10 d.rast lsat7_2002_20 d.rast lsat7_2002_30 d.rast lsat7_2002_40
We can easily query the spectrum for individual points:
d.what.rast lsat7_2002_10,lsat7_2002_20,lsat7_2002_30,lsat7_2002_40
Histogram of a channel:
d.histogram lsat7_2002_10
Create RGB view on the fly, true and false color:
d.rgb b=lsat7_2002_10 g=lsat7_2002_20 r=lsat7_2002_30 d.rgb b=lsat7_2002_70 g=lsat7_2002_20 r=lsat7_2002_30 d.rgb b=lsat7_2002_70 g=lsat7_2002_50 r=lsat7_2002_30 d.rgb b=lsat7_2002_70 g=lsat7_2002_50 r=lsat7_2002_10
Check thermal channel (encoded to fit into 0.255 data range):
- http://landsathandbook.gsfc.nasa.gov/handbook/handbook_htmls/chapter11/chapter11.html
- Look up LMIN/LMAX: "Table 11.2 ETM+ Spectral Radiance Range watts/(meter squared * ster * µm)"
- we won't go into details here, r.mapcalc would be used to convert to Kelvoin/degree Celsius.
# look at encoded thermal channel d.rast lsat7_2002_61
NDVI as example for map algebra:
r.mapcalc "ndvi = 1.0 * (lsat7_2002_40 - lsat7_2002_30)/(lsat7_2002_40 + lsat7_2002_30)" d.rast.leg ndvi r.colors ndvi color=ndvi d.rast.leg ndvi
Show selected NDVI "classes":
d.rast ndvi val=0.3-1.0 d.rast ndvi val=-1.0--0.1 d.rast ndvi val=0.0-0.2 d.rast ndvi val=0.0-0.3
Look at panchromatic channel, compare resolution:
g.region rast=lsat7_2002_80 -p d.erase -f d.rast lsat7_2002_80 d.zoom d.rast lsat7_2002_30 d.rast lsat7_2002_80
Image Classification
Set region setting to red image:
g.region rast=lsat7_2002_30 -p
Create a group
i.group group=lsat7_2002 subgroup=lsat7_2002 \ input=lsat7_2002_10,lsat7_2002_20,lsat7_2002_30,lsat7_2002_40,lsat7_2002_50,lsat7_2002_70
Unsupervised classification
Generate unsupervised statistics
i.cluster group=lsat7_2002 subgroup=lsat7_2002 sigfile=lsat7_2002_sig classes=10 reportfile=lsat7_2002.txt
# look at report file gedit lsat7_2002.txt
Assign pixels to classes, check quality of assignment:
i.maxlik group=lsat7_2002 subgroup=lsat7_2002 sigfile=lsat7_2002_sig class=lsat7_2002_class reject=lsat7_2002_reject
# false color composite d.mon x0 ; d.font Vera d.rgb b=lsat7_2002_70 g=lsat7_2002_50 r=lsat7_2002_10 # classification result d.mon x1 ; d.font Vera d.rast.leg lsat7_2002_class # rejection map d.mon x2 ; d.font Vera d.rast.leg lsat7_2002_reject # rejection histogram d.mon x3 ; d.font Vera d.histogram lsat7_2002_reject
![]() |
![]() |
![]() |
![]() |
Play with different cluster separations:
i.cluster group=lsat7_2002 subgroup=lsat7_2002 sigfile=lsat7_2002_sig classes=10 reportfile=lsat7_2002.txt separation=1.5 i.maxlik group=lsat7_2002 subgroup=lsat7_2002 sigfile=lsat7_2002_sig class=lsat7_2002_class2 reject=lsat7_2002_reject2 d.mon x4 d.rast.leg lsat7_2002_class2 d.rast.leg lsat7_2002_reject2 d.rast.leg lsat7_2002_reject d.rast.leg lsat7_2002_reject2 d.rast.leg lsat7_2002_reject d.rast.leg lsat7_2002_reject2 d.rast.leg lsat7_2002_class2
Compare to RGB:
d.rgb b=lsat7_2002_70 g=lsat7_2002_50 r=lsat7_2002_10 d.rast lsat7_2002_class2 cat=1 -o d.rgb b=lsat7_2002_70 g=lsat7_2002_50 r=lsat7_2002_10 d.rast lsat7_2002_class cat=1 -o d.rgb b=lsat7_2002_70 g=lsat7_2002_50 r=lsat7_2002_10 d.rast lsat7_2002_class cat=1 -o
We do a 3rd attempt with more classes
i.cluster group=lsat7_2002 subgroup=lsat7_2002 sigfile=lsat7_2002_sig classes=15 reportfile=lsat7_2002.txt i.maxlik group=lsat7_2002 subgroup=lsat7_2002 sigfile=lsat7_2002_sig class=lsat7_2002_class3 reject=lsat7_2002_reject3 d.rast.leg lsat7_2002_class3
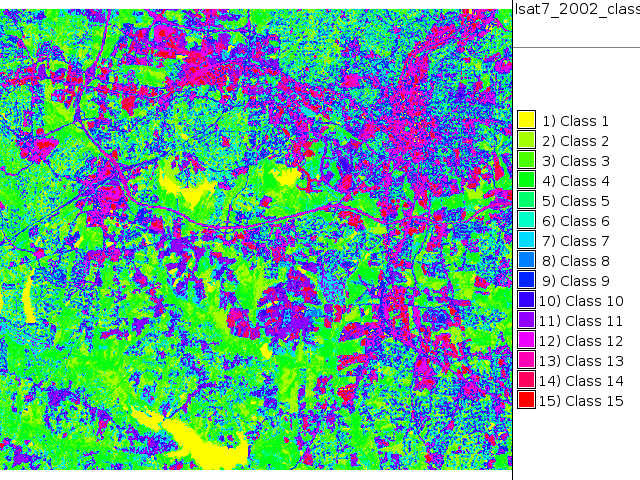
Supervised classification
Launch GUI for digitizer:
g.gui wxpython
Now digitize training areas of water, asphalt, forest, uncovered soil on RGB composite in GUI. Digitize polygons/centroids with attributes. The advantage: we know what the classes are.
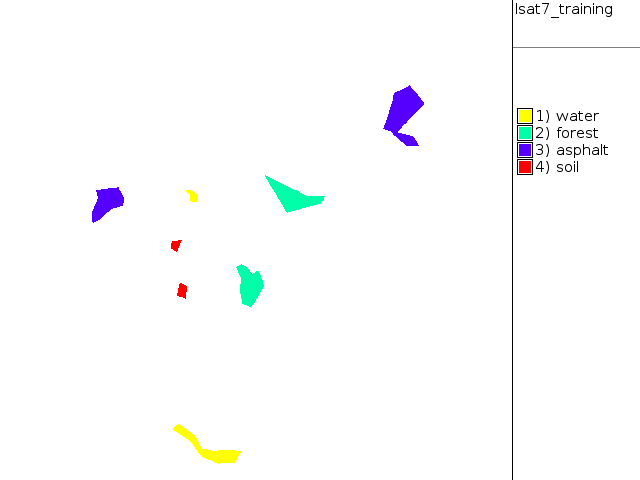
Assign also numerical ID for each class in new column
v.db.select lsat7_training v.db.addcol lsat7_training col="id integer" v.db.select lsat7_training v.db.update lsat7_training col=id where="name = 'water'" val=1 v.db.update lsat7_training col=id where="name = 'forest'" val=2 v.db.update lsat7_training col=id where="name = 'asphalt'" val=3 v.db.update lsat7_training col=id where="name = 'soil'" val=4 v.db.select lsat7_training
Transform vector training map to raster model:
g.region vect=lsat7_training align=lsat7_2002_10 -p v.to.rast in=lsat7_training out=lsat7_training use=attr col=id labelcol=name --o d.mon x0 d.rast.leg lsat7_training
Generate statistics from training areas
i.gensigset group=lsat7_2002 subgroup=lsat7_2002 sig=lsat7_2002_smap training=lsat7_training
Perform supervised classification
i.smap group=lsat7_2002 subgroup=lsat7_2002 sig=lsat7_2002_smap out=smap
Colorize:
r.colors smap rules=- << EOF
1 aqua 2 green 3 180 180 180 4 brown EOF
d.rast.leg smap d.rgb b=lsat7_2002_10 g=lsat7_2002_20 r=lsat7_2002_30
![]() |
![]() |
Vectorize result:
r.to.vect smap out=smap feat=area d.rgb b=lsat7_2002_10 g=lsat7_2002_20 r=lsat7_2002_30 d.vect smap type=boundary col=red
That's it :)
Object-based classification
Created based on Summary of object-based classification possibilities in GRASS GIS (June 2014). Feel free to merge this with other content or with different page.
- Moritz's steps and the main discussion: Object-based image classification in GRASS (nabble link)
- Moritz's steps in a nice guide by Martin, in Czech with images (English Google-translation)
- Alternative notes by Moritz: Classification of segments (nabble link)
- Pietro's steps and tools (nabble link)
- Another discussion about what variables to use: Object-based classification (nabble link)
- The source code of the classification tools (for inspiration in writing your own scripts):
- v.class.ml: http://svn.osgeo.org/grass/grass-addons/grass7/vector/v.class.ml/ (Pietro's tool)
- v.class.mlpy: http://svn.osgeo.org/grass/grass-addons/grass7/vector/v.class.mlpy/ (Vaclav's tool)
- i.mlpy.py: http://svn.osgeo.org/grass/sandbox/turek/i.mlpy.py (Stepan's tool)
- List of Python machine learning libraries:
- scikit-learn, http://scikit-learn.org/ (used by v.class.ml)
- mlpy, http://mlpy.sourceforge.net/ (used by v.class.ml, v.class.mlpy and i.mlpy)
- Pandas, http://pandas.pydata.org/
- Milk, http://luispedro.org/software/milk
Further reading classification with GRASS
- Micha Silver: Analyzing acacia tree health in the Arava with GRASS GIS
- Perrygeo: Impervious surface deliniation with GRASS
- Dylan Beaudette: Working with Landsat Data
- Dylan Beaudette: Canopy Quantification via Image Classification
- Segmentazione o classificazione d'immagini con GRASS (in italiano)
- Di Palma, F.; Amato, F.; Nolè, G.; Martellozzo, F.; Murgante, B. 2016: A SMAP Supervised Classification of Landsat Images for Urban Sprawl Evaluation. ISPRS Int. J. Geo-Inf. 2016, 5, 109. PDF: http://www.mdpi.com/2220-9964/5/7/109