NLCD Land Cover
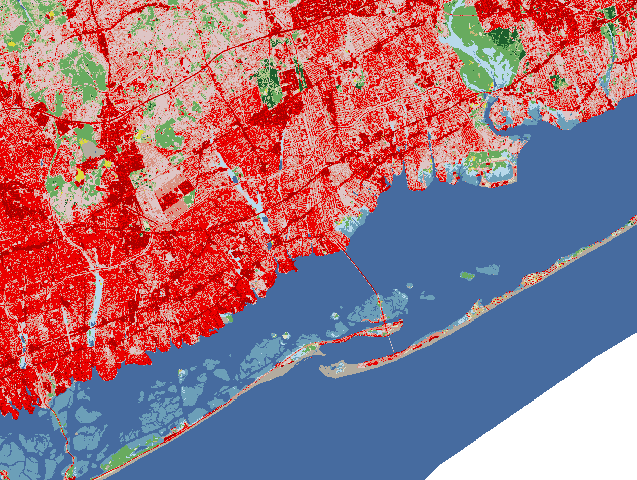
About
- Raster grid covering the Continental US at 30 meter resolution
From the Spearfish dataset's landcover.30m history file:
- National Land Cover Data Set NLCD, U.S. Geological Survey (USGS)
- The NLCD was compiled from Landsat TM imagery (circa 1992) with a spatial resolution of 30 meters supplemented by various ancillary data (where available). The satellite images were analyzed and interpreted by using very large, sometimes multi-State, image mosaics (that is, up to 18 Landsat scenes). Because a relatively small number of aerial photographs were necessarily conducted from a broad spatial perspective. Furthermore, the accuracy assessments correspond to Federal Regions, which are groupings of contiguous States. Thus, the reliability of the data is greatest at the State or multi-State level. The statistical accuracy of the data is known only for the region.
Obtaining NLCD data
North Carolina
- Download site: National Land Cover Database (NLCD) - 30m
- https://www.lib.ncsu.edu/gis/nlcd
- download: NC_NLCD2019only.zip
- https://www.lib.ncsu.edu/gis/nlcd
Spearfish
The classic Spearfish GRASS sample dataset contains a landcover.30m raster map which is an excerpt from an older version of the NLCD dataset.
- Download site: https://www.mrlc.gov/data
- Data is provided in ERDAS Imagine .img format.
- As of April 2019 the zipped dataset is a 1.4 GB.
- Create an Albers location
- this can also be done automatically with the Location Wizard using the downloaded .img file.
Here are the values for the CONUS dataset:
name: Albers Equal Area proj: aea datum: wgs84 ellps: wgs84 lat_1: 29.5 lat_2: 45.5 lat_0: 23 lon_0: -96 x_0: 0 y_0: 0 towgs84: 0,0,0,0,0,0,0 no_defs: defined
Importing
North Carolina
You can easily import (r.import) the NC_NLCD2019only dataset into the NC sample dataset mapset by the following steps
mkdir NC_NLCD2019only
cd NC_NLCD2019only
unzip /path/to/NC_NLCD2019only.zip
r.import input=nc_nlcd2019 output=nc_nlcd2019
Spearfish
Rather than import the entire 16GB raster image, just make a virtual link to it:
r.external in=NLCD_2016_Land_Cover_L48_20190424.img out=NLCD_2016_Land_Cover_L48_20190424 title="Land Cover 2016"
Then use g.region to zoom to your area of interest and r.mapcalc to extract a subset
g.region -p n= s= e= w= res=30 align=NLCD_2016_Land_Cover_L48_20190424
r.mapcalc "NLCD.extract = NLCD_2016_Land_Cover_L48_20190424"
Categories
These can be applied with the r.category module.
0:Unclassified 11:Open Water 12:Perennial Snow/Ice 21:Developed, Open Space 22:Developed, Low Intensity 23:Developed, Medium Intensity 24:Developed, High Intensity 31:Barren Land 41:Deciduous Forest 42:Evergreen Forest 43:Mixed Forest 52:Shrub/Scrub 71:Herbaceous 81:Hay/Pasture 82:Cultivated Crops 90:Woody Wetlands 95:Emergent Herbaceous Wetlands
Colors
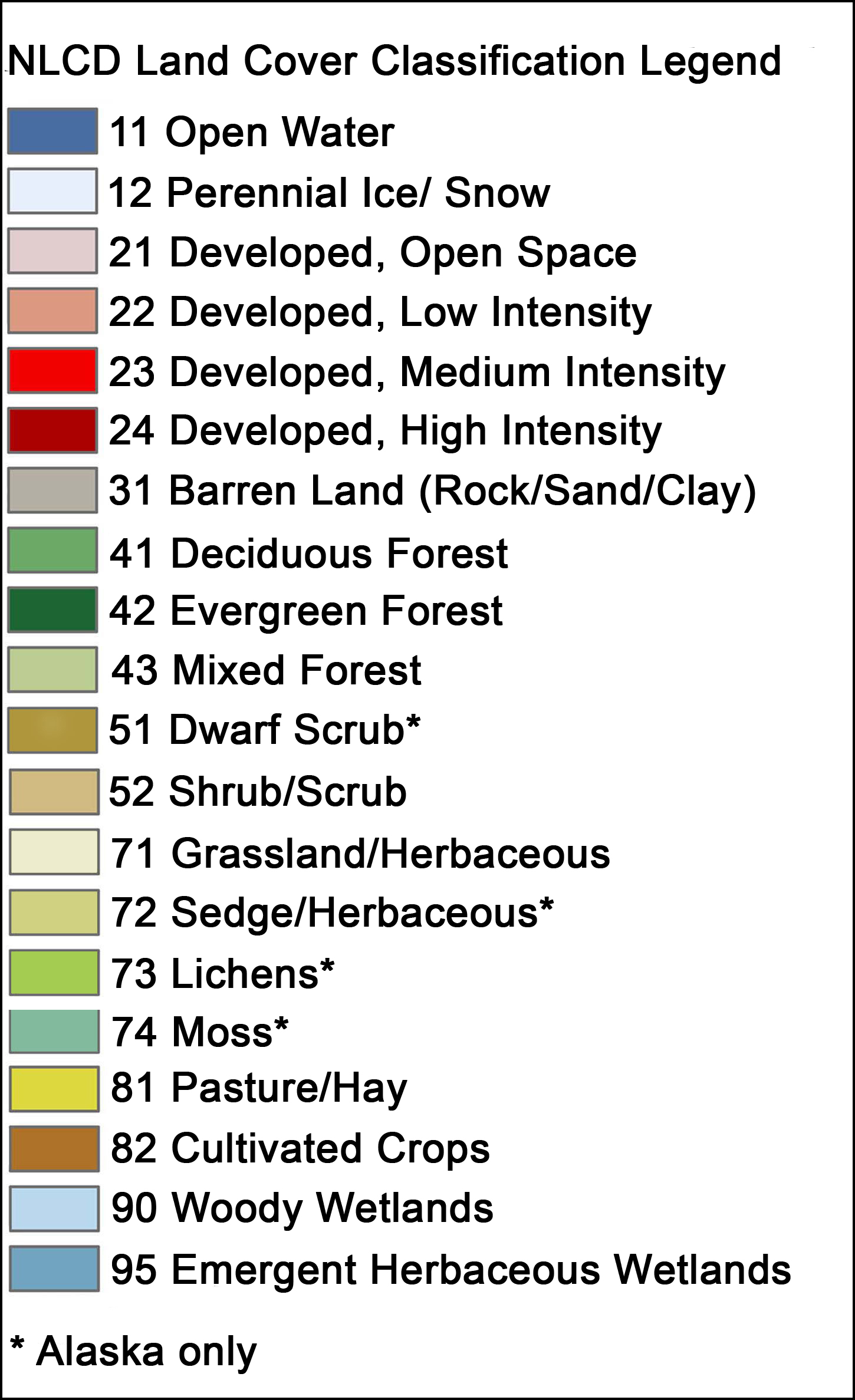
These should load in automatically, but if they don't here are the official colors which you can load in with the r.colors module's rules= option:
11 70:107:159 12 209:222:248 21 222:197:197 22 217:146:130 23 235:0:0 24 171:0:0 31 179:172:159 41 104:171:95 42 28:95:44 43 181:197:143 52 204:184:121 71 223:223:194 81 220:217:57 82 171:108:40 90 184:217:235 95 108:159:184
Legend
Use the -n and -c flags of d.legend to limit the legend to named categories.
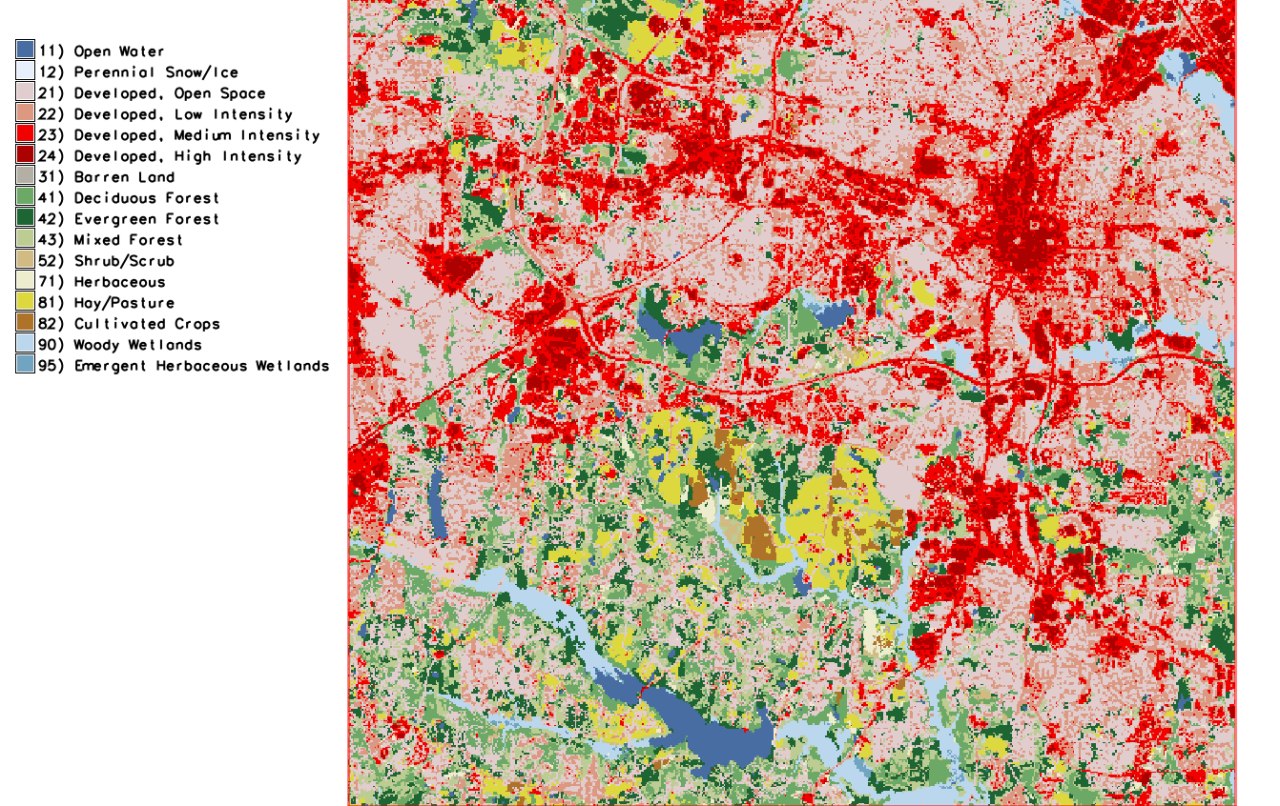
Manning's n
For using as a basis for Mannings n friction coefficients, you can make a virtual reclass map with r.reclass. The module will only reclass as integers so the table below is reclassed into parts per ten-thousand. Crop your area of interest with r.mapcalc and divide by 10000.0 at the same time to get the Manning's n number.
Actual values for your location will vary widely, the following is simply a starting point.
0 = 0 Unclassified 11 = 0250 Open Water 12 = 0220 Perennial Snow/Ice 21 = 0400 Developed, Open Space 22 = 1000 Developed, Low Intensity 23 = 0800 Developed, Medium Intensity 24 = 1500 Developed, High Intensity 31 = 0275 Barren Land 41 = 1600 Deciduous Forest 42 = 1800 Evergreen Forest 43 = 1700 Mixed Forest 52 = 1000 Shrub/Scrub 71 = 0350 Herbaceous 81 = 0325 Hay/Pasture 82 = 0375 Cultivated Crops 90 = 1200 Woody Wetlands 95 = 0700 Emergent Herbaceous Wetlands
References:
- Ven Te Chow, 1959 (Corvallis)
- USGS's Guide for Selecting Manning's Roughness Coefficients for Natural Channels and Flood Plains
- Kalyanapu, Alfred & Burian, Steve & Mcpherson, Timothy. (2009). Effect of land use-based surface roughness on hydrologic model output. Journal of Spatial Hydrology. 9. 51-71.
- Liu, Zhu & Merwade, Venkatesh & Jafarzadegan, Keighobad. (2018). Investigating the role of model structure and surface roughness in generating flood inundation extents using 1D and 2D hydraulic models. Journal of Flood Risk Management. e12347. 10.1111/jfr3.12347.
- Bunya, et al. (2010). A High-Resolution Coupled Riverine Flow, Tide, Wind, Wind Wave, and Storm Surge Model for Southern Louisiana and Mississippi. Part I: Model Development and Validation. Monthly weather review 138.2: 345-377.